Artificial Intelligence and machine learning tools as a whole have taken important steps in development within the last decade. Specifically, AI tools have been getting more sophisticated in their learning and human-like decision-making.
Optimal Pricing
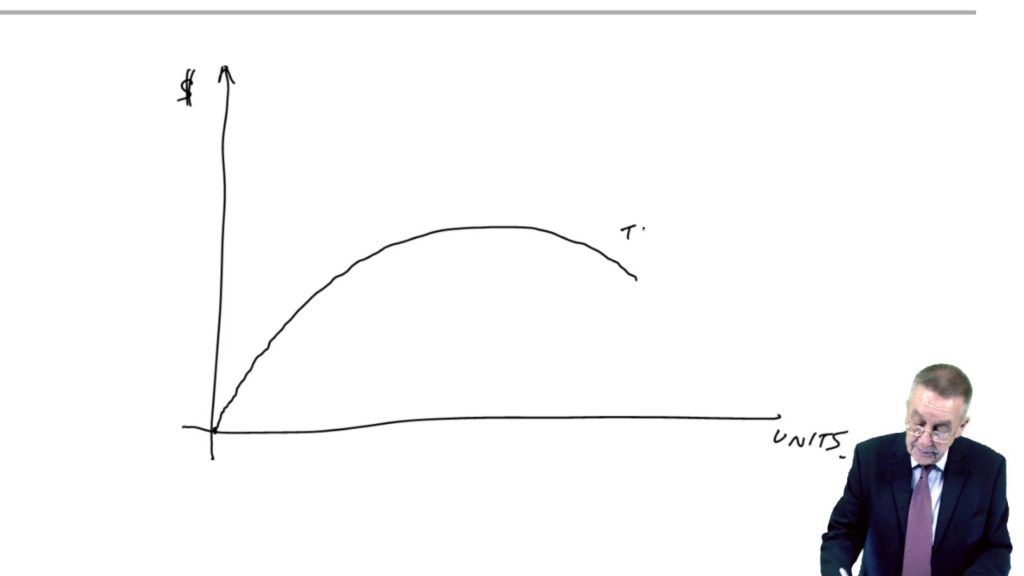
In the past, competitive pricing was almost purely human-oriented. Those in charge of pricing analytics would check their competitors’ prices and respond accordingly, taking into account other factors such as demand or brand loyalty. The key consideration here, especially with online shopping, is that customers are usually comparing prices in multiple stores before deciding to buy. Forrester Consulting found that in 2014, 81% of surveyed Americans compared online prices before buying.
Seeing as competitive pricing is nearly a must in the consumer-oriented market, doing such pricing analytics by hand is not only time consuming but also limited in scope. Because there are so many factors to consider—demand, brand power, competitor prices, seasonality—it isn’t easy to get a full picture of optimal pricing decisions. With the advent of AI in retail market analysis, a load of extensive research and uncertain decision-making is taken off of employees’ shoulders.
In a way, competitive pricing AI takes all the information that would have been researched by people and combines the data into one decision-making paradigm. These algorithms can analyze things like competitor pricing or behavior (promotion patterns, the regularity of price changes, etc.), and a retailer’s essential products to draw a transparent pricing system. They can detect patterns that humans would have trouble with; one such example would be product matching, wherein the machine learning tools recommend products that are more likely to be bought in tandem. Another example is pricing simulations, where different pricing scenarios are tested (you can dive deeper into this subject in this Competera’s blog post).
Artificial Intelligence can be implemented in practically any industry for optimal pricing—even for something like an online pet store. In short, AI has completely changed the way retailers look at dynamic pricing, and turned the human guessing game into a science.
Customer Support
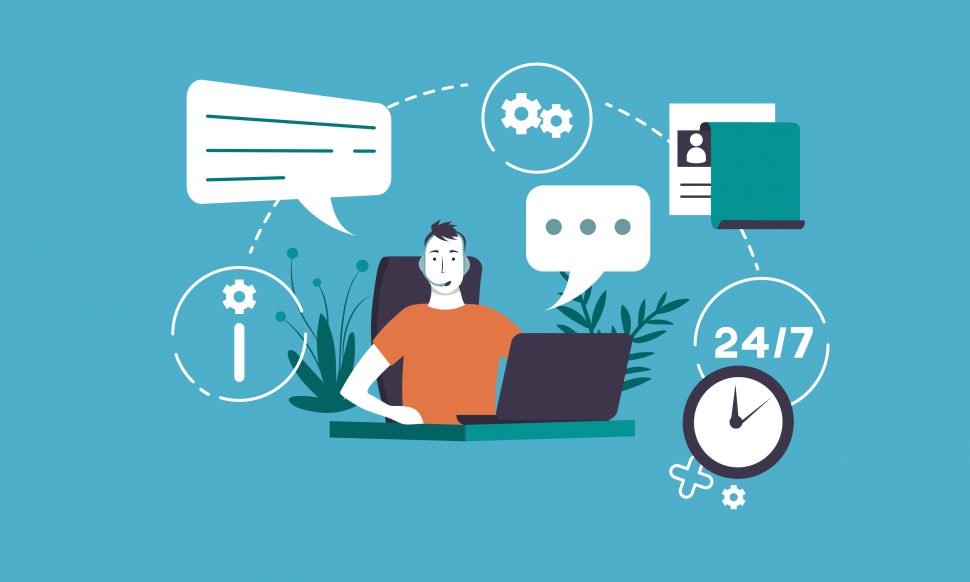
Advanced technologies and software have been advancing customer service for decades. Keeping your customers happy is one of the keys to a successful and prosperous business and is vital to building a loyal consumer base. Thus, using the newest technology to get the edge in customer service is a recurring theme in well-liked retailers.
A large portion of customer satisfaction is contingent on the speed at which any issues are resolved; if your support team has limited hours or a waiting list, customers could be kept waiting. This is why chatbots are essential in the digitized retail market. They are up 24/7 and can often help resolve the user’s issue without the need for a representative. If the bot can’t solve the customer’s problem or question, it can always transfer the subject and some necessary information regarding it to a human rep.
Some chatbots are even advanced enough to offer advice and learn the types of problems customers often report. The North Face’s AI bot is capable of providing advice based on user input, effectively acting as an in-store representative. Similarly, Lidl’s chatbot Margot helps buyers pick out a wine, and boasts the ability to mimic natural speech. All in all, chatbots are a vital auxiliary, if not vital, tool for around-the-clock customer support.
Personalized Experience
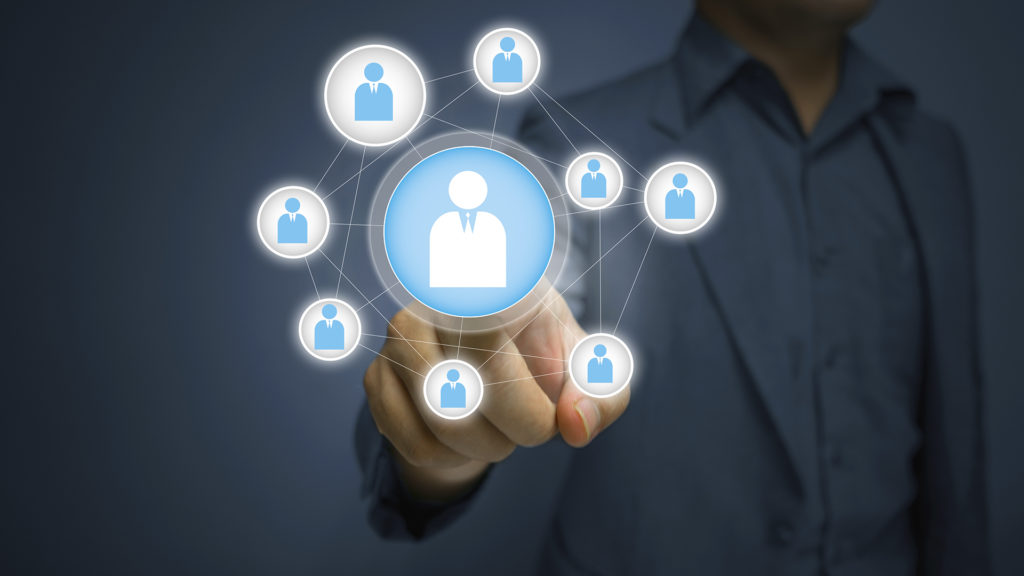
Machine learning has revolutionized targeted advertising and suggestions due to the volume of customer data available nowadays. Every action, from search history to browsing patterns, is carefully analyzed to tailor shopping or even browsing experience. The most prevalent form of this is probably targeted advertising; while it can come off as an invasion of privacy at times, the ads we see online are largely based on what these algorithms believe we are likely to click on or be interested in.
If your browsing history is filled with product pages for headphones or searches like “best noise-canceling earbuds”, you are reasonably likely to see ads for headphones soon enough. It’s almost like having someone next to you throw out suggestions based on your browsing patterns. Sometimes, their ideas might be neat and useful.
A similar personalization of experience can be seen on sites like Netflix. Based on what you’ve seen and enjoyed in the past, it’ll suggest shows or movies the AI thinks you’ll like. The reason AI works so well in this regard is that it analyzes more than basic categories, and can extrapolate real-time insights into a customer’s preferences. Another example would be Amazon—products are suggested to you based on your browsing and purchase history.
Customer Outreach
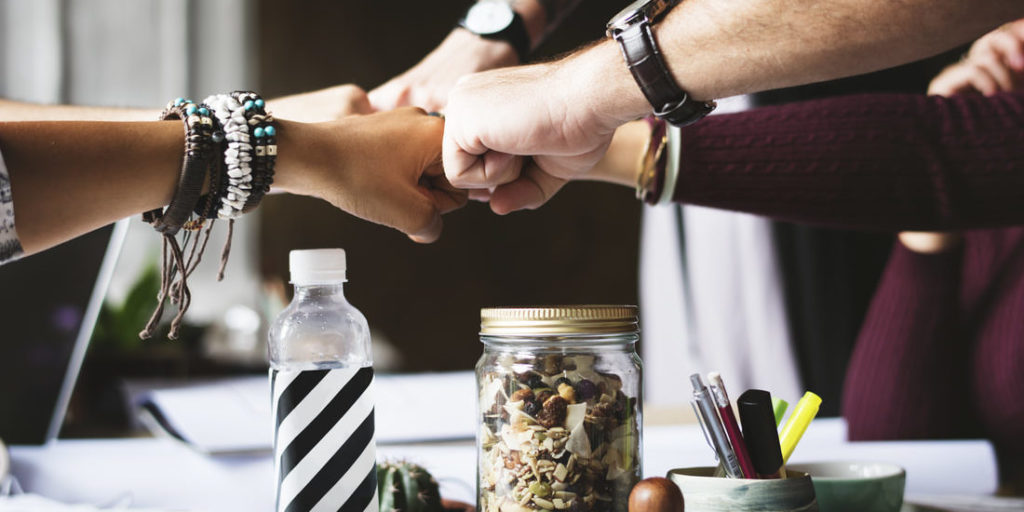
Before email became the dominant means of communicating with consumers, businesses would have to rely on telephone and post for marketing purposes. Of course, these methods are far more expensive and effectively obsolete in the retail sphere. In the early days of email’s prevalence in marketing, though, businesses had to rely on pre-written text for all customers—sort of like a TV commercial.
Since then, email marketing has taken huge strides and often boasts higher conversion rates than social media marketing and similar means. One key component in the evolution of email is its personalization; in 2016, Starbucks revamped its rewards system in a push for more engagement. They went from cycling through 30 email variations to sending 400,000 personalized emails per week.
The personalization of email marketing increases the likelihood that consumers will be interested in reading an email, and, as Starbucks proved, can improve sales and customer engagement. At its core, it also adds a particular “human” aspect to a very calculated and automated marketing strategy.
Conclusion
Artificial Intelligence is vastly reshaping the way retailers make business decisions and connect with their consumers. At the start, technology was more of a means for people to optimize their work. In recent years, though, AI has been gaining the knack of identifying patterns and connecting with consumers, bridging the gap between human intelligence and cold, calculating machinery.